Breakthrough Proof Clears Path for Quantum AI – Overcoming Threat of “Barren Plateaus”
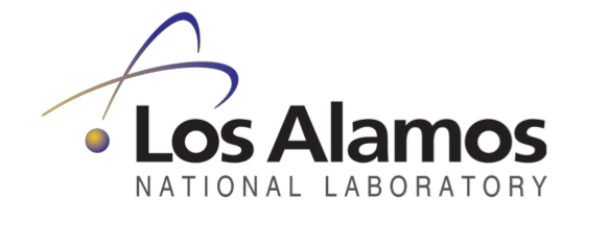
(SciTechDaily) A novel theorem developed at Lawrence Livermore National Labs demonstrates convolutional neural networks can always be trained on quantum computers, overcoming threat of ‘barren plateaus’ in optimization problems.
Convolutional neural networks running on quantum computers have generated significant buzz for their potential to analyze quantum data better than classical computers can. While a fundamental solvability problem known as “barren plateaus” has limited the application of these neural networks for large data sets, new research from LANL overcomes that Achilles heel with a rigorous proof that guarantees scalability.
“The way you construct a quantum neural network can lead to a barren plateau—or not,” said Marco Cerezo, coauthor of the paper titled “Absence of Barren Plateaus in Quantum Convolutional Neural Networks,” published recently by a LANL team.
Performing computation using quantum-mechanical phenomena such as superposition and entanglement.</div>”>quantum computing, quantum machine learning, and quantum information at Los Alamos. “We proved the absence of barren plateaus for a special type of quantum neural network. Our work provides trainability guarantees for this architecture, meaning that one can generically train its parameters.”
As an artificial intelligence (AI) methodology, quantum convolutional neural networks are inspired by the visual cortex. As such, they involve a series of convolutional layers, or filters, interleaved with pooling layers that reduce the dimension of the data while keeping important features of a data set.
These neural networks can be used to solve a range of problems, from image recognition to materials discovery. Overcoming barren plateaus is key to extracting the full potential of quantum computers in AI applications and demonstrating their superiority over classical computers.
Until now, Cerezo said, researchers in quantum machine learning analyzed how to mitigate the effects of barren plateaus, but they lacked a theoretical basis for avoiding it altogether. The Los Alamos work shows how some quantum neural networks are, in fact, immune to barren plateaus.
“With this guarantee in hand, researchers will now be able to sift through quantum-computer data about quantum systems and use that information for studying material properties or discovering new materials, among other applications,” said Patrick Coles, a quantum physicist at Los Alamos and a coauthor of the paper.
Many more applications for quantum AI algorithms will emerge, Coles thinks, as researchers use near-term quantum computers more frequently and generate more and more data—all machine learning programs are data-hungry.